Table Of Content
In the "Modify Design" menu, users can modify factors, replicate design, randomize design, renumber design, fold design, and add axial points. To change the factors, click the "Modify factors" radio button and then "Specify" to see the following options menu. Minitab 15 Statistical Software is a powerful statistics program capable of performing regressions, ANOVA, control charts, DOE, and much more. Minitab is especially useful for creating and analyzing the results for DOE studies. It is possible to create factorial, response surface, mixture, and taguchi method DOEs in Minitab.
Checking Residuals Using Minitab's Four in One Plot
In addition, the complexity of delivering multiple combinations of components can be reduced by using a fractional factorial design (Collins et al., 2009), which reduces the number of different component combinations per the number of factors used. While more research on IC interactions is surely needed, our research has consistently found such interactions (Cook et al., 2016; Fraser et al., 2014; Piper et al., 2016; Schlam et al., 2016). Thus, it might be difficult in many cases to assume conditions that would justify the use of a fractional factorial design. A 2×4 factorial design is a type of experimental design that allows researchers to understand the effects of two independent variables on a single dependent variable. Interactions occur when the effect of an independent variable depends on the levels of the other independent variable. As we discussed above, some independent variables are independent from one another and will not produce interactions.
Achieving the Right Component Comparisons
Factorial designs are the basis for another important principle besides blocking - examining several factors simultaneously. We will start by looking at just two factors and then generalize to more than two factors. Investigating multiple factors in the same design automatically gives us replication for each of the factors.
Basic Elements of RCT and Factorial Designs
All rights are reserved, including those for text and data mining, AI training, and similar technologies. For all open access content, the Creative Commons licensing terms apply. The last four column vectors belong to the A × B interaction, as their entries depend on the values of both factors, and as all four columns are orthogonal to the columns for A and B. For example, imagine that researchers want to test the effects of a memory-enhancing drug. Participants are given one of three different drug doses, and then asked to either complete a simple or complex memory task. The other approach is a weighted analysis, where you weight the observations according to the inverse of their variance.
Minitab DOE Example
Indeed, even without the use of special assessments, investigators might correlate the number of ICs a person receives (regardless of type) to outcomes. For the vast majority of factorial experiments, each factor has only two levels. For example, with two factors each taking two levels, a factorial experiment would have four treatment combinations in total, and is usually called a 2×2 factorial design. In such a design, the interaction between the variables is often the most important. This applies even to scenarios where a main effect and an interaction are present.
3.8. Example bar graphs¶
This will allow you to determine the effects of temperature and pressure while saving money on performing unnecessary experiments. By the traditional experimentation, each experiment would have to be isolated separately to fully find the effect on B. This would have resulted in 8 different experiments being performed. Note that only four experiments were required in factorial designs to solve for the eight values in A and B. A factorial experiment allows for estimation of experimental error in two ways.
V. Chapter 5: Experimental Research
It should be quite clear that factorial design can be easily integrated into a chemical engineering application. Many chemical engineers face problems at their jobs when dealing with how to determine the effects of various factors on their outputs. For example, suppose that you have a reactor and want to study the effect of temperature, concentration and pressure on multiple outputs. In order to minimize the number of experiments that you would have to perform, you can utilize factorial design.
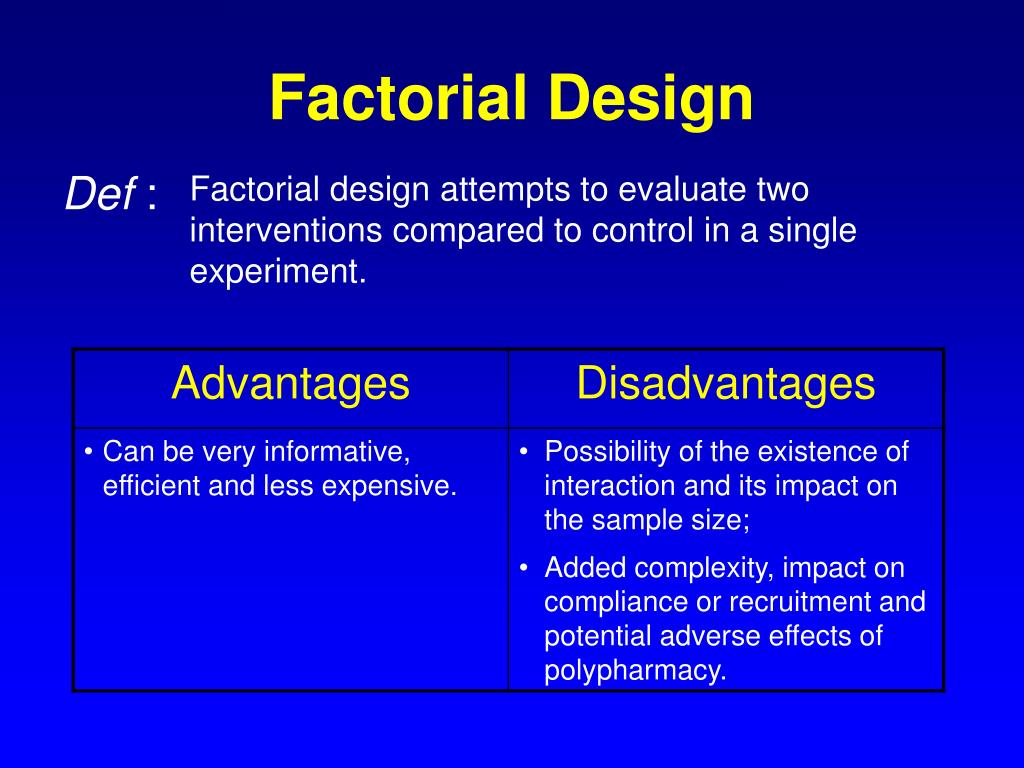
We began with the full model with all the terms included, both the main effects and all of the interactions. From here we were able to determine which effects were significant and should remain in the model and which effects were not significant and can be removed to form a simpler reduced model. We can perform a two-way ANOVA to formally test whether or not the independent variables have a statistically significant relationship with the dependent variable. The primary way of doing this is through the statistical control of potential third variables. Instead of controlling these variables by random assignment or by holding them constant as in an experiment, the researcher measures them and includes them in the statistical analysis. Consider some research by Paul Piff and his colleagues, who hypothesized that being lower in socioeconomic status (SES) causes people to be more generous [PKCote+10].
The Pareto charts are bar charts which allow users to easily see which factors have significant effects. A null outcome situation is when the outcome of your experiment is the same regardless of how the levels within your experiment were combined. From the example above, a null outcome would exist if you received the same percentage of seizures occurring in patients with varying dose and age. The graphs below illustrate no change in the percentage of seizures for all factors, so you can conclude that the chance of suffering from a seizure is not affected by the dosage of the drug or the age of the patient. Treatment combinations are denoted by ordered pairs or, more generally, ordered tuples.
Let's look at another example in order to reinforce your understanding of the notation for these types of designs. Here is an example in three dimensions, with factors A, B and C. Below is a figure of the factors and levels as well as the table representing this experimental space. In this type of design, one independent variable has two levels and the other independent variable has four levels. Data from 2x2 designs is often present in graphs like the one above. An advantage of these graphs is that they display means in all four conditions of the design.
Also, sometimes when you have unequal variance you just have a couple of bad outliers, especially when you only have one or a few observations per cell. In this case it is difficult to the distinguish whether you have a couple of outliers or the data is heteroscedastic - it is not always clear. All three of these residuals versus the main effects show same pattern, the large predicted values tend to have larger variation. A transformation - the large values are more variable than smaller values. Well, when we fit a full model it only has one observation per cell and there's no pure way to test for residuals. But when we fit a reduced model, now there is inherent replication and this pattern becomes apparent.
However, some challenges are of particular relevance to factorial designs. What this points out is the importance of distinguishing what is a block factor, and which are the treatment factors when you have a multifactor experimental design. If there is interaction and it is significant, i.e. the p-value is less than your chosen cut off, then what do we do?
It is up to researchers to interpret and label the factors and to explain the origin of that particular factor structure. For example, one reason that extraversion and the other Big Five operate as separate factors is that they appear to be controlled by different genes [PDMM08]. The difference between red and green bars is small for level 1 of IV1, but large for level 2.
Don't forget about the “scientist” in your Data Scientist title (Part 2) - Towards Data Science
Don't forget about the “scientist” in your Data Scientist title (Part .
Posted: Thu, 06 Jan 2022 08:00:00 GMT [source]
So while the largest main total effect value in one set of experiments may have a value of 128, another experiment may have its largest main total effect value be 43. Suppose you have two variables \(A\) and \(B\) and each have two levels a1, a2 and b1, b2. You would measure combination effects of \(A\) and \(B\) (a1b1, a1b2, a2b1, a2b2). Since we have two factors, each of which has two levels, we say that we have a 2 x 2 or a 22 factorial design. Typically, when performing factorial design, there will be two levels, and n different factors. A main effects situation is when there exists a consistent trend among the different levels of a factor.
Simplest reaction time analysis for experimental psychology? - ResearchGate
Simplest reaction time analysis for experimental psychology?.
Posted: Fri, 29 Mar 2019 07:00:00 GMT [source]
For instance, while a real world application of a treatment might involve the administration of only two bundled ICs (counseling + medication), a factorial experiment might involve 6 or more ICs. Such effects would be manifest in interactions amongst components (e.g., the effectiveness of a component might be reduced when it is paired with other components) or in increased data missingness. Moreover, if higher order interactions are not examined in models, researchers will not know if an intervention component is intrinsically weak (or strong) or is meaningfully affected by negative (or positive) interactions with other factors.
No comments:
Post a Comment